Q&A: Is agentic AI ready to take over the contact center?
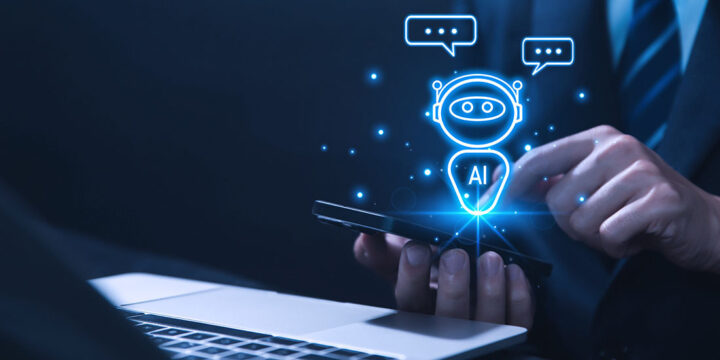
As we enter 2025, agentic AI has quickly become the latest buzzword to capture the public imagination. In the contact center, it promises a not-too-distant future where AI agents can take on more complicated decision-making and directly replace some expensive human workflows in the process.
However, like the grand visions cast about generative AI and multimodal AI that came before, it can be difficult to tell how much of the hype for agentic AI is real (and ready for implementation today) and how much of it is still hypothetical value.
To better understand what agentic AI means for the customer experience today, tomorrow, and next year, we sat down with our Director of AI Solutions, Aaron Schroeder, for an honest conversation about its strengths and weaknesses as part of an AI-enabled contact center strategy.
Questions we cover in this Q&A:
- What is agentic AI, and how does it differ from some of the other recent AI buzzwords like generative AI and multimodal AI?
- Why is agentic AI generating so much buzz right now?
- How close are we to unlocking agentic AI use cases in real customer experience environments?
- What challenges are holding back agentic AI?
- If true agentic AI will be here in 2026, what should organizations do now to lay the groundwork in 2025?
For starters, let’s level-set on agentic AI. What is agentic AI, and how does it differ from some of the other recent AI buzzwords like generative AI and multimodal AI?
Aaron Schroeder: Let’s start with generative AI. Generative AI has been around for a while at this point. As a refresher, it refers to the ability of AI to ingest, analyze, and produce new structured or unstructured data — such as human conversations or computer code — using deep-learning models derived from a training data set or large language model (LLM). Think of it like the foundation on which multimodal and agentic AI use cases are built. In both of these newer forms of AI, generative AI is a critical component.
From there, multimodal AI came first. In the context of the customer experience, multimodal AI allows brands to point AI at use cases that may contain a mix of modalities such as images, voice, text and video. It emerged with the first broadly accessible and useful models in early 2024, but it has grown into a powerful capability that we now use with our clients all the time to help them automate more complicated customer queries. For example, auto insurance clients use multimodal AI to assess automotive claim photo submissions from their customers, dealers, or insurance agents — accelerating the end-to-end submission process and allowing live agents to focus on other aspects of claim fulfillment.
Agentic AI takes this foundation of capabilities and then combines it with rapidly improving thinking and reasoning models to equip AI to handle an entire end-to-end workflow and verify its work along the way. In theory, this will allow AI agents to act on behalf of live agents or participate in tasks across an agent’s typical workflow, with less or even no oversight — generating meaningful contact center cost savings in the process.
Why is agentic AI generating so much buzz right now?
AS: With each new AI technology leap, businesses get closer to realizing their vision of an AI-powered customer experience, but they haven’t quite reached the holy grail yet. To fully realize the cost savings potential of AI, fully independent AI agents will always be the end goal.
Think about it like this:
As new LLMs like DeepSeek drive down the cost of using AI models by reducing the cost to complete the underlying agent tasks, businesses are incentivized to find more use cases for it. Agentic AI holds the key to unlocking more complex interactions that might otherwise represent a significant cost to the business’ bottom line.
AI that generates ROI
Start with our guide to AI-enabled CX transformation to explore the business case for strategic AI adoption.
How close are we to unlocking agentic AI use cases in real customer experience environments?
AS: Much like multimodal AI a year ago, agentic AI likely will not experience widespread adoption until 12 to 16 months from now. According to the latest Gartner AI report, less than 1% of enterprise applications were using agentic AI in 2024.
That said, 1% adoption might be a bit misleading. If we break AI use cases down into five distinct levels of agency, some of them are already viable today.
- Do as I say—This first level of agency includes use cases where AI has been preprogrammed to respond in a specific, defined way. This has been around for a while and can be found in use cases like robotic process automation (RPA).
- Research and tell me—This second level is also widely available today. The multimodal AI auto claims process I shared earlier is one example of this level of agency in the wild.
- Recommend to me—This level of agency builds on “research and tell me” by taking multiple complex inputs, synthesizing them, and making a recommendation to a human in the loop (HITL) based on those inputs.
But, when we’re talking about agentic AI, we’re really talking about the final two levels of AI agency. These last two levels unlock true AI-enabled decision-making that enables the cost-efficient vision many organizations have for their contact center.
- Decide and tell me about it—Minimal human oversight, beyond process and outcome auditing at the supervisor level.
- Do it and don’t bother me—Little to no human oversight, as AI agents take care of various processes and customer queries entirely on their own.
These levels are largely not ready for implementation today, due to several different challenges at both the LLM and business process levels.
What challenges are holding back agentic AI?
AS: Let’s start with three big challenges. First and foremost, there are the legal concerns. As you increase the level of agency and decision-making you delegate to an AI agent, you also accept the risks associated with those decisions. Depending on the industry, incorrect decisions could lead to lawsuits, PR nightmares, and poor outcomes for the end customer.
Additionally, these final two agentic AI levels require comprehensive training data that many organizations have not documented or curated for AI usage today. Unlike the AI that came before, agentic AI needs access to relevant knowledge bases, conversation data, business process context, as well as APIs to all the systems where the AI agent will need to “act” on its decisions.
Finally, AI models are frozen in time. In other words, agentic AI today would make real-time decisions based on slightly outdated data. When we think about human decision-making, we’re constantly being bombarded by new information that refines our decisions. Timeliness of data is a significant hurdle that needs to be cleared to help agentic AI make sound decisions.
If true agentic AI will be here in 2026, what should organizations do now to lay the groundwork for adoption in 2025?
AS: In addition to establishing a set of AI governance principles you can use to organize and regulate your business’s AI adoption over time, there are a couple specific ways you can prepare your organization for agentic AI.
Most importantly, start by experimenting with the first three levels of agentic AI now. These capabilities are ready today and can already deliver meaningful ROI across the contact center. The learnings you gain from experimenting with AI now will help focus and strengthen your approach over time.
To be ready for levels four and five, you need to master your business processes across the customer experience. For every type of interaction, what are the potential actions a live agent takes and what are the tools, applications, and data needed to take that action? Mapping out these decision trees and actions is crucial information to feed agentic AI solutions so that they can achieve the right outcomes.
Taking this one step further, implementing a business process management (BPM) approach to strategically analyze existing processes for optimization opportunities is one step businesses can take right now to begin to spotlight which current processes and workflows might be ripe for AI to take over. By starting with a business process audit, organizations can prepare themselves for rapid agentic AI adoption when the time comes.
Ready to explore AI use cases that deliver real impact?
Our AI Value Labs are designed to help you do just that. Contact one of our AI experts to get started.
Join our mailing list
Receive exclusive updates on the latest CX trends, events, and solutions.