Only you can prevent robot uprisings: Our advice on enhancing your CX with AI
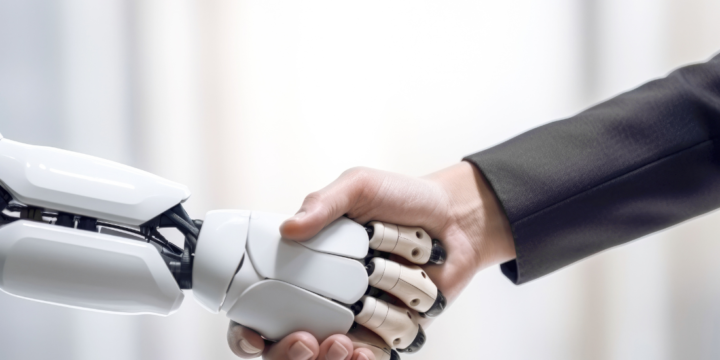
Right now, generative artificial intelligence (AI) tools like ChatGPT are making headlines — and in some cases, being used to write those headlines — leading to excitement and speculation about what the future of work might look like. But there is also a lot of anxiety around trusting important decisions to these generative tools. These anxieties are misplaced.
Over the years, plenty of writers and artists have imagined what an automated world populated by sentient AI would look like, reflecting the hopes and fears of that specific time period. When you watch old movies or TV shows and see the techno-optimism of the Jetsons or the hostile takeover of the machines in the Matrix, you’re really seeing a time capsule of whatever humanity was most concerned about at the moment.
AI isn’t sentient yet. Just like our stories about AI reflect the hopes and fears that we have, AI is still just a reflection of us, trained by large language models to react in limited ways to specific situations using human knowledge. It’s still humanity at the center.
And THAT is what we should really be concerned about.
What is Generative AI?
Generative Artificial Intelligence is a term for algorithms that can be used to generate new content. While AI models can recognize patterns in data that would be too massive and complicated for a human to do efficiently, Generative AI can recognize and classify similarities in a vast pool of data and then generate new content based on those patterns. This content can include text, audio, code, images, simulations, and even videos, and it can be made very quickly.
AI doesn’t make its own decisions, but it does make “decisions” using human input — faster, more efficiently, and at scale. Unfortunately, this also means it can quickly replicate human biases, prejudices, and mistakes much faster, more efficiently, at scale, and with less oversight.
But there’s no putting the genie back in the bottle now. As AI pervades more and more aspects of our lives and how we make decisions, it’s something we can’t afford to ignore.
And we shouldn’t have to. AI, when utilized responsibly, has the capacity to change how we work. AI can help us make better decisions, make us, smarter, healthier, and more efficient. The possibilities are endless.
Why AI is Ideal for Customer Experience
Contact centers are responsible for the bulk of customer experience and are the main point of contact customers have with a brand. Agents in these centers are often expected to juggle up to eight different channels to provide service on the customer’s channel of choice and tie together a story from disparate sources of data. Between the attrition rates at contact centers, long wait times, the great resignation, and other challenges in the industry, a tool that can make decisions and respond faster and more efficiently seems like a dream come true.
And it can be — when utilized correctly.
What AI Can and Can’t Do for CX
The expectation customers have today is hyperresponsiveness: being known, valued, and helped in the right way at the right time.
When it comes to traditional customer experience (CX) delivery through a customer contact center with human agents, most brands have an extremely high standard. In every experience and every interaction, brands are aiming for perfection. We know we’re not perfect — but it’s the ideal we aim for and the baseline we measure by. When we fall short, we iterate processes, retrain, and try to learn how we can improve.
There are things that generative AI does quite well. And there are things that it does… OK.
AI still isn’t providing a human touch. AI is great at repetitive, mundane tasks, or providing relevant information almost instantaneously. What this means is that AI is great at augmenting human workers, empowering live agents with the tools and data they need to become more efficient and more effective, and helping a human agent anticipate why someone might be calling, or providing background gathered from across different channels to help the agent piece together what might be happening.
How to Enhance the Human Touch with AI
The first bullet on Google’s Recommended AI Practices is to use a human-centered design approach: “The way actual users experience your system is essential to assessing the true impact of its predictions, recommendations, and decisions.”
If you want to have excellent AI-enhanced CX, but don’t want to be responsible for the robot uprising, we’ve written a guide on how to enhance your customer experience with AI using a human-centric approach. With a nod to Isaac Asimov’s Three Laws of Robotics, here are the Three Laws of Human-Centered Design in AI-Driven CX.
The Three Laws of Human-Centered Design in AI-Driven CX
1. Think Responsibly
“A robot may not injure a human being or, through inaction, allow a human being to come to harm.”
Ethics can feel like a loaded word. But when you’re talking about a paradigm-shifting tool, it’s an important clarification. How can you ensure that you are using AI ethically? Here are some considerations:
- Data Lineage - You’re given a data set, but do you know where it came from or how it came about? Did it capture someone’s biases when it was being made? Biases don’t have to be malicious or obvious to have an effect. As an example, did someone collecting names at a conference misspell names they were less familiar with, or did everyone type in their own names on the form?
- Input Bias - Is your ability to intake information reflecting your expectations, and rejecting input from outside those expectations? Do you need to reconsider what your expectations are? I’ve seen forms where the minimum character input for a name was three letters. Let’s say your name is Li. How do you even fill out the form? When your dataset is excluded from the start, how likely are you to trust the outcome?
- Privacy – There is no single correct model and balancing privacy against utility will have to be measured in each individual scenario, but there are some best practices. Try to avoid using sensitive data when less sensitive data will suffice and anonymize and aggregate data. I recommend Google’s page on privacy best practices for further reading.
- Reinforcing Prejudices – Some companies have found in the past that when you include data inputs like gender, models perform better in determining credit eligibility. You want the most accurate data possible, right? What’s the harm in reflecting the way the world actually is — even if it feels unfair or uncomfortable?
STOP. It’s ridiculous to think you can impartially observe without influencing the outcome when you are actively reinforcing the wrong outcome. You are reinforcing the wrong thing, and you can wreak long-lasting damage. You are not an impartial observer: You are responsible for creating the kind of world you want to live in.
- Diverse Perspectives - Bring diverse perspectives to the table and think about the entire process holistically. What is this going to influence? What are possible unintended outcomes, good or bad? What will the downstream effects be?
2. Consider Overlooked Angles & Consequences
“A robot must obey orders given it by human beings except where such orders would conflict with the First Law.”
Everyone’s favorite flying saucer-shaped autonomous vacuum, the Roomba, has an open-source code for its decision engine, making it possible to “hack their brain.” One hack that someone created for it was to optimize efficiency — every time it bumped into something or had to turn, that would be counted as a negative. The longer it could go without doing that was a positive. She theorized that it would be more efficient if it spent less time turning around or stopping from bumping.
The result? The Roomba started driving backward. Roombas don’t have sensors or bumpers on their back, so the little robot eventually “learned” to drive backward to maximize rewards.
This obviously didn’t achieve the intended objective, but it technically maximized the reward score; it achieved the objective to the Roomba’s understanding. Technically correct! The best kind of correct.
What is your reward mechanism? Are you sure?
The Transportation Security Administration (TSA) has looked to AI screening to reduce discomfort for airline travelers, but their highest-performing solution for improving the experience of the majority of passengers may have to be rejected. Why? Because it would flag everyone as “not a threat.”
Statistically, that AI program would be nearly 100% accurate. There were 253 million airline passengers in the United States in 2019. I’m too nervous to search for exact numbers on how many attempted hijackings were thwarted that same year on my work account, but it could probably be counted on two hands or fewer. The vast majority of people that go through a security checkpoint in an airport are not a threat. If you tell AI to be as accurate as possible when presented with those numbers, rounding down to 0 is the most logical solution.
But this is a case where you want zero mistakes. Discovering a potential threat is arguably worth a few false positives and extra pat-downs. According to Google’s recommended practices, “Ensure that your metrics are appropriate for the context and goals of your system, e.g., a fire alarm system should have high recall, even if that means the occasional false alarm.”
When you’re setting up your AI outcomes, you need to consider the consequences of it being wrong. If you can’t be 100% accurate, what kind of inaccuracy can you settle for? What is the consequence of a false negative versus a false positive?
The best way to combat this is through continuous evaluation and evolution. Even if you think you’re getting the kinds of outcomes you wanted, are you measuring the downstream effects of those outcomes? What has the conversation been around updating based on these evaluations? Whether it’s a policy change or updates, your AI can only be as effective as the data it’s given. Does the data you used yesterday still have the same impact today?
Speaking of continuous evolution…
3. Keep a Human in the Loop
“A robot must protect its own existence as long as such protection does not conflict with the First or Second Law.”
Don’t give up at your first mistake. Identifying an issue in your outcome or process isn’t the end — in fact, it’s an opportunity. If you’re discovering a bias in your field or column, or that you’re producing inadequate outcomes, it’s just that: a bad field, a bad column, and an opportunity to clean your data or reassess your process. It does not mean you need to scrap the whole idea. One violation existing in a vacuum doesn’t mean you scrap the entire product, feature, or department. (Do make sure it’s in a vacuum, though.)
This is a good reason to have a process in place from the beginning, before issues arise, for assessing the root cause of bad information and evaluating where you can deploy changes. And that’s why it’s important to keep a human in the loop.
Keeping a human in the loop provides guardrails, ensuring that your AI solution is giving you the outcomes you want and the solutions you want. Humans can also provide a, well, human touch since human emotions are more complicated than 90 discrete buckets.
Enhancing AI with a Human Touch
While generative AI technology is new and exciting and endlessly promising, it can’t be the magic cure to all your CX problems. You still have to put in the work to understand what your customers want, where the gaps are, and what you can do to overcome those gaps. If you want to add AI to your toolkit, you still have to answer two questions: What outcomes are you trying to drive today in your capability set? And, what is preventing you from accomplishing your CX goals?
AI can be a useful tool in providing best-in-class CX, but the complex challenges of setting it up correctly and ensuring that it can provide the outcomes you want are much easier when faced with a partner. A partner like TTEC Digital, who understands the intersection of AI and CX and the current technology platforms that exist, can help you be more thoughtful in your decision and in your ability to execute your best possible customer journey.
Are you ready for customer-worthy AI?
Take the assessment to clarify your AI goals and learn how you can use AI to create customer-worthy experiences.
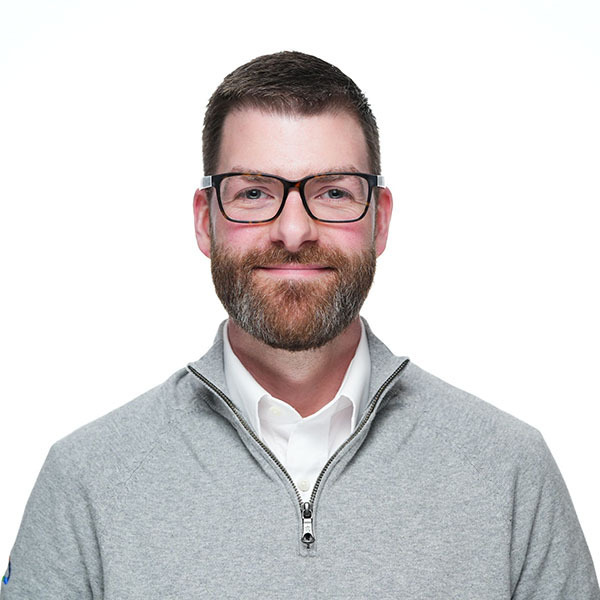
About the Author
Aaron Schroeder
Director, AI SolutionsAaron aids clients in leveraging AI to enhance speed, consistency, and innovation in customer experience.
Read moreJoin our mailing list
Receive exclusive updates on the latest CX trends, events, and solutions.