Why choosing the best LLM for your AI-enabled contact center matters less than you think
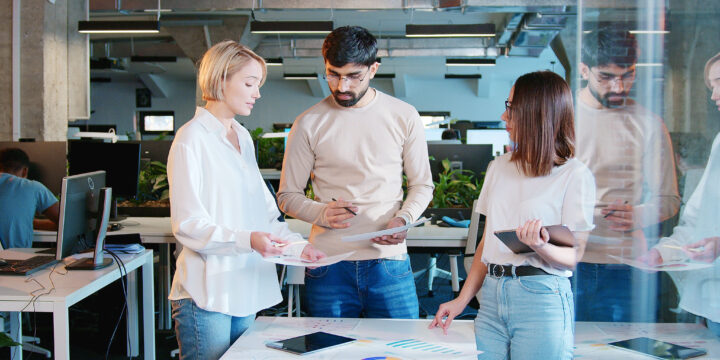
Since ChatGPT propelled generative AI onto the scene more than a year ago, the AI landscape has continued to change rapidly. From a growing list of potential use cases in the contact center, to a plethora of tools and technology to make it happen, it’s no wonder many organizations feel a little overwhelmed by the prospect of implementing AI solutions and policies.
So far, a handful of different applications have risen to the top. Conversation summarization, virtual agents, AI-enabled knowledge management, and conversation intelligence — all of these solutions offer powerful advantages to the contact centers that deploy them strategically within their larger customer experience strategy. Yet these decisions tend to come downstream of more foundational AI decisions.
Which AI use cases make the most sense for your customer experience?
Read the guide to explore how AI and automation can factor into contact center ROI.
As a starting point, we’re seeing many organizations take on a deeper exploration into Large Language Models (LLMs) and attempt to proactively select the foundation model or models that support their AI initiatives going forward. On the surface, this makes sense, as the number of LLMs has grown dramatically in the last year. With this in mind, the question that I keep hearing from IT and data science teams is this:
We want to have the best LLM. Which model do you recommend — both in the short term and the future?
My short answer: The LLM you choose today matters less than you think.
Here are two reasons why this is true.
Reason #1: Over the last year, we’ve seen how fast the consensus around the #1 LLM can change and evolve with each new update.
According to one Elo ranking leaderboard for LLMs, Lmsys.org, GPT-4 held the top rating in May 2023. In April of this year, after countless changes to the leaderboard, Claude 3 Opus sat in first place. For context, Claude 3 – or even Claude 1 for that matter – did not yet exist when the May 2023 rankings came out.
My point is, for the past year, we’ve seen the LLM landscape change in unpredictable ways. The minute an organization commits to an LLM, it’s already on borrowed time. Within weeks, it will be usurped by the new model on the block.
Reason #2: The gaps we’re seeing between LLMs at the top of the leaderboards are shrinking.
As my last point illustrates, new LLM versions and updates are coming out in fast succession. I believe this is a sign that we’re rapidly approaching the top of the S-curve in this regard.
For example, Google released a research paper in April that claimed it was able to give LLMs the ability to work with text of infinite length. Essentially Google’s technique makes it possible to extend the LLM’s context window, with only slight tradeoffs in memory and compute required. This means that one of the major constraints preventing greater context window accuracy, the limit to how much content could be consumed, has largely been solved. After all, you can’t really expand a context window beyond infinite, can you?
At a macro level, we’re seeing entire classes of LLMs reaching similar expected ceilings in performance. GPT-4 already achieves 95.3% accuracy when tested on HellaSwag or commonsense reasoning. Gemini Ultra now earns a 94.4% on Grade School Math and 90.4% on MMLU (language understanding).
While new benchmarks may need to be devised to track other changes and targeted capabilities, this shows that many of the general capabilities are trending toward a peak. Moving forward, advancements will likely come in specialized areas, rather than broad improvements across the baseline metrics.
Ultimately, the leveling out of LLM performance at the top of the leaderboard is good news. For one it reduces the magnitude of your initial LLM choice. But more importantly, it helps to re-position contact center AI implementations on the aspects that will actually drive the most long-term value to your operation – things like, flexibility, ease of deployment, and integration into existing processes.
How to approach AI implementation to maximize long-term success
Keeping in mind that LLMs are not the same differentiator they were even a year ago, there are two principles organizations should focus on when it comes to implementing AI.
Principle #1: Act now
Knowing that LLMs are increasingly similar from a performance standpoint, it’s important to focus on value. That is, organizations need to focus their attention on the technologies and strategies that will enable them to achieve their desired outcomes.
For starters, each day your organization remains AI-free, your competitors are rapidly honing their AI-enabled customer experiences to deliver the effortless experiences that win over loyalty and wallet share.
So, how do you act fast, without acting too fast?
Oftentimes, a pilot project is the right approach to take. With a pilot, you can identify upfront success metrics and begin to capture results and measure value on a smaller scale.
Principle #2: Innovate often
Just because LLMs have begun to plateau for now, doesn’t mean they’ll remain that way forever. There will almost certainly be additional jumps in performance across new media (and multimodal media) as our collective AI journey continues.
Additionally, the way customers want to engage with automated and AI-enabled tools will also change. For years, chatbots were seen as an inconvenience to customers who felt they would be unable to meet their needs. Modern AI is changing this perception, which leads to the question: Where else will AI change public perception in the years to come?
For this reason, it’s better to invest in AI expertise (whether internal or external) to help you navigate the evolution of AI, rather than one cutting-edge LLM and set of AI solutions now. Launching an AI initiative is just the beginning. Expert guidance is the only way to stay on the pulse of AI best practices and ensure you’re continuing to make the incremental process, operations, and people changes over time that will drive big value.
Ultimately, investing in the ongoing delivery aspects that will surround your initial AI solution, rather than focusing on the nuts and bolts of your AI modeling, will generate much more profound growth in your ROI.
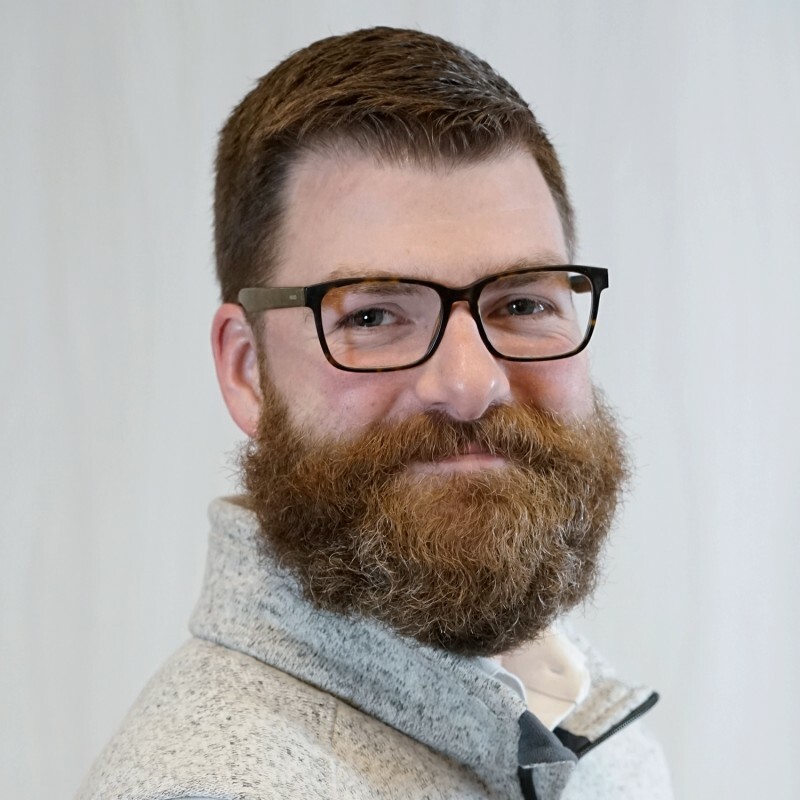
About the Author
Aaron Schroeder
Director, AI SolutionsAaron aids clients in leveraging AI to enhance speed, consistency, and innovation in customer experience.
Read moreJoin our mailing list
Receive exclusive updates on the latest CX trends, events, and solutions.
Looking for an AI expert contact center partner?
Learn more about our SurroundCX™ Managed Services to see how TTEC Digital approaches long-term AI-enabled customer experience transformation planning.